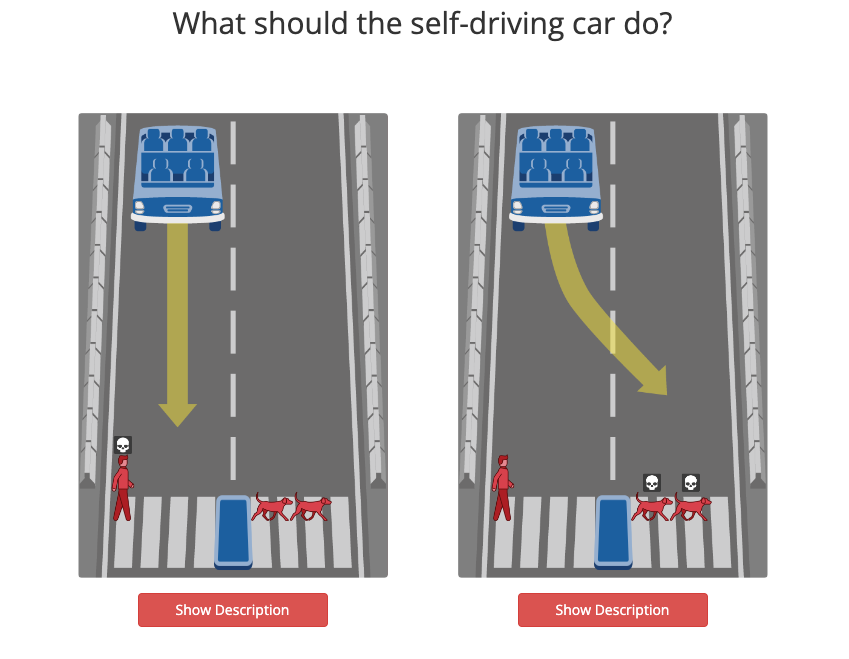
How to build better AI products with user research | by Claire Jin | Feb, 2024
[ad_1]
The concept of computer programs simulating human thought and decision-making, known as Artificial Intelligence (AI), is not new. It dates back to the 1950s. For years now, we’ve been surrounded by products leveraging Machine Learning (ML). It determines what we see on social media, the GPS systems that find the best travel routes, and the recommendation engines on e-commerce websites that suggest products adapted to our preferences.
Yet, we’re witnessing a leap forward driven by advancements in computing power and the availability of extensive datasets. These factors have enabled breakthroughs in generative AI, a subset of AI focusing on creating new content and solutions, and Large Language Models (LLMs), designed to interpret and generate human language. Our relationship with technology is undergoing a profound transformation, unveiling new possibilities that were once beyond our imagination.
With everyone excited about AI, a fear of missing out (FOMO) is driving companies to embed AI into every product feature. This can lead to a technology-centric approach, overshadowing a fundamental goal of product development: to create solutions that genuinely solve user problems and meet their needs.
In fact, rushing into AI-powered solutions without understanding user needs, clearly defining problems, and evaluating AI’s appropriate applications often leads to disappointing results. In some cases, especially when AI solutions are used without sufficient safeguards in sensitive areas like healthcare, the consequences can be outright dangerous, posing risks to user privacy, safety, and well-being.
As UX professionals, it’s part of our role to tackle these topics, and I firmly believe that user research has never been more essential.
Levels of AI integration
From a UX perspective, at its most common, AI enhances traditional software functionalities such as autocomplete or predictive analysis. Google’s autocomplete feature, for instance, intelligently predicts search queries from minimal user input, offering a more efficient and customized search experience.
In these cases, both user researchers and users often find themselves in familiar territory. Sometimes, AI’s presence is so subtle that users might not even recognize it’s there. The focus of user research here is on understanding how these AI enhancements impact user interactions and perceptions of the product’s value.
Then, AI can become a driver of user interaction. Consider, for instance, chatbots or voice recognition systems. A familiar example is the voice assistant in our cellphones, like Apple’s Siri on the iPhone, which can perform tasks such as sending messages based on our voice commands.
Here, user research is centered around how users adapt to these new forms of interaction, assessing their intuitiveness, efficiency, adoption and overall satisfaction.
The interface can also integrate AI to automate or completely redefine workflows, marking a significant leap in the human-machine relationship. Generative AI in logo design is a simple example: it autonomously performs steps that were traditionally human-led such as sketching initial ideas, experimenting with shapes, colors, typography, etc. Here is a logo I created using DALL·E for ‘Explorer’s Edge’, a fictitious adventure travel agency.
Jakob Nielsen, in his article AI: First New UI Paradigm in 60 Years, highlights the generative AI transformation:
“With the new AI systems, the user no longer tells the computer what to do. Rather, the user tells the computer what outcome they want.”
User research at this stage explores new questions, including trust in AI’s decision-making capabilities, defining the scope of automation, and ensuring a balance between machine operation and user control. It also explores the emotional connections users form with AI systems.
This paradigm shift requires UX teams and user researchers to continuously adapt and evolve their approaches to address new challenges, and we’ll explore some of them in this article.
Embracing the unseen: the ‘Wizard of Oz’ method
The ongoing transformation in AI usage is leading us from static interfaces to proactive, personalized assistants, finely tuned to individual preferences, contexts, and habits and enabling end-to-end experiences. These new concepts need to be tested, especially since development is costly and prototyping, particularly with generative AI, presents significant challenges.
This is precisely where the ‘Wizard of Oz’ method comes into play. In this user research method, users interact with a fake automated interface that is actually controlled by a human behind the scenes. It shifts from closed scenarios, featuring predefined responses ideal for structured exploration, to open ones, where responses are defined in real-time to gain a deeper understanding of user expectations.
I started using the ‘Wizard of Oz’ back when we didn’t have easy access to models like GPT. This technique allowed us to simulate a real-time chatbot on a website and a voice assistant for phone-based customer support.
We gained essential insights, such as pinpointing the specific interaction modes that resonated with different audiences, the precise moments when users sought a human touch, and the tones of voice that struck a chord.
This method also proved invaluable in our collaboration with engineers. It enabled us to steer the development of machine learning models with a precision that only first hand user insights can offer. Iterations that might have taken months and heavily strained budgets with real ML models were instead completed in just days with a strong focus on the user. And let’s admit it, playing the wizard is an enjoyable experience!
Uncovering and adapting to context and new mental models: longitudinal studies
Innovative AI features often unfold their true potential over time, extending well beyond the initial phase of user discovery and interaction. Consider how user habits evolve from the 1st to the 10th, or even the 100th use of an AI product. These transitions can sometimes involve a learning curve, which we need to study and anticipate. They can also involve the development of emotional bonds with the product, for instance, through interactions with AI assistants that offer personalized responses.
As AI products introduce new mental models, understanding and tracking the shift in users’ perceptions, expectations, and emotional engagements become indispensable. Longitudinal studies offer a powerful approach to track these changes over time.
Longitudinal user testing is a practical option and relatively easy to implement. This method involves conducting user tests at various stages of a product’s lifecycle, with the same group of users. It’s highly advisable to collect user data in advance to create scenarios that are as personalized and contextually relevant as possible. This method allows for observation of the user experience’s evolution with product changes and as users grow more accustomed to it.
Diary studies are also very effective for AI products. In this approach, participants maintain a diary — often digital — logging their interactions with a product or service over a period of time. This method yields rich, qualitative data on the evolving user experience, marked by its challenges, frustrations, and delightful moments. Such insights provide critical clarity on the users’ real-world context.
For instance, in a project where I worked on an intelligent home assistant equipped with voice recognition, one basic functionality was selecting a playlist for the household member in the room. The diary studies brought to light unforeseen scenarios, like when multiple family members with different preferences were present or when the user had guests over. They also underscored the influence of the time of day and the user’s mood on their interactions with the product, highlighting the need for AI systems to adapt to these dynamic human factors. These insights helped to adapt the product to the complexities of real-life situations.
For those interested in exploring further AI diary studies, I recommend the Nielsen Norman Group article on information foraging with ChatGPT, Bard, and Bing Chat.
Enhancing continually AI models: user feedback loops
Just like with any product, it’s essential to continuously gather feedback on user interactions through various methods, such as analytics, A/B testing, in-app surveys, and session replays.
This is especially true for AI products from the beginning of the development cycle as the model evolves continuously. Initiating pilot testing early on is essential. It can start with alpha testing, where internal teams rigorously examine the AI models. Such an in-depth review by diverse organizational expertise allows to identify and rectify critical functionality and accuracy concerns. Following alpha testing, beta testing allows to introduce the AI product to a selected group of real users, broadening the scope of feedback. This phase focuses in understanding how the product performs in the complexity of day-to-day use, gaining first-hand user-centered insights.
One of the specific aspect of AI products is their capacity for immediate adjustments. User feedback, embedded in the product, can directly train and refine machine learning algorithms, with each interaction serving as a new data point that enhances the AI’s accuracy and responsiveness to user preferences. When implementing these feedback mechanisms, it’s essential to strike a balance to prevent overwhelming the user.
Let’s examine a few real-world applications of existing feedback mechanisms. They often include thumbs-up/thumbs-down ratings, queries about the relevance or usefulness of results, and options for users to exclude or report content.
Netflix has a ‘rate’ feature, where users can influence recommendations by selecting options like ‘not for me,’ ‘I like this,’ or ‘Love this.’
In the Deezer music streaming app, users can express their dislike for a track or artist, asking the system not to recommend similar options in the future.
In an AI chatbot like ChatGPT, users can provide feedback on generated content, compare different responses, or select the best one.
These feedback results can be analyzed in conjunction with other user research methods, for instance, examining user logs or conducting follow-up interviews, to gain deeper insights and a more comprehensive context.
The rapid advancements in AI models open new opportunities for user research, suggesting the need for innovative real-time feedback loop formats.
Navigating the ethical terrain: the role of user research in AI trust and responsibility
As AI becomes more prevalent, the tension between quick integration and adoption and ethical challenges arises. Various organizations and companies are working on AI Principles to guide responsible development, for instance, UNESCO and the AI Alliance, which promotes safe and responsible AI through open innovation. Tackling issues such as bias, transparency, safety, moral concerns, and user control from the start of AI product development is key. User research can help teams navigate these challenges and mitigate ethical debt and negative social consequences.
A particular focus must be given to the biases within training datasets. Such biases have the potential to significantly affect AI performance and user experience, often perpetuating and even amplifying existing inequalities. For instance, the Elevate Black Voices project, a research collaboration between Google and Howard University demonstrates how incorporating a wider range of voices and perspectives can significantly enhance speech recognition technologies for all users. Similarly, in recruitment practices where AI is increasingly utilized, careful examination of biases is essential to prevent discrimination. User researchers bring important skills in ensuring data representativeness and ethical integrity, adapting established frameworks and employing essential tools like consent forms and data management policies for responsible data collection.
User research methods like user tests, interviews, focus groups, and surveys are useful in ensuring continuous ethical assessment during product development. Specialized research experiments can also be used. The Moral Machine experiment, for instance, focuses on moral decisions in autonomous vehicles, aiming to gather human perspectives on these dilemmas. This experiment revealed a global trend to prioritize saving humans over pets, sparing larger groups over individuals, and protecting children and pregnant women. The experiment also unveiled specific cultural disparities. For example, the preference to spare younger characters rather than older characters is much higher for Latin America and France, than for countries such as Japan, Indonesia, Pakistan and Saudi Arabia. These are considerations that need to be discussed.
Keeping a broad perspective becomes increasingly important as products serve global markets. Numerous studies available online can shed light on societal attitudes toward AI. The graph below, taken from the Ipsos Global Views on AI report 2023, illustrates notable variations in trust toward AI, underscoring cultural differences that can influence user acceptance. The study displays significant disparities, with emerging markets and individuals under the age of 40 demonstrating notably higher levels of trust. This can inform and refine user research strategies, ensuring products are relevant across different cultural contexts.
User research also helps in understanding the impact of AI decisions, such as the consequences of inaccuracies. Anticipating and monitoring potential errors, dark patterns and the negative impacts of AI systems allows for informed design choices. In my case, the stakes varied greatly from one project to another. In e-commerce, a misaligned recommendation might only be mildly annoying or irrelevant to the customer. However, in critical sectors like energy, inaccuracies in a predictive model for managing network parameters could pose significant safety hazards. Comprehensive user research can help to mitigate risks and establish guardrails. Ethnographic studies, in particular, can provide insights into how AI is used in complex real-world scenarios, offering a more complete understanding of user interactions. For example, by conducting studies in the energy sector, we found that even processes, which appeared easily automatable, actually depended on unconnected systems and needed manual input. This underscored the need to allow manual adjustments to the AI model’s parameters for accurate alignment.
[ad_2]
Source link